Amplified’s large language model (LLM) powered search beats previous best scores
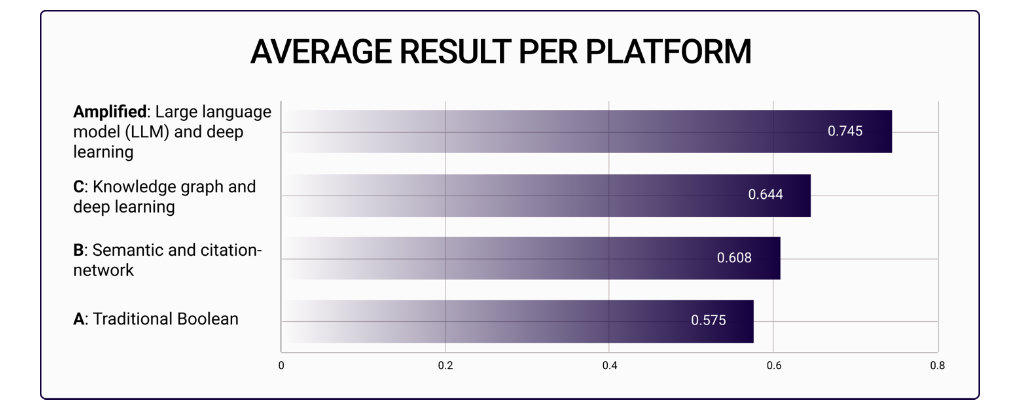
Since 2020, Riahi Patents has been closely following the evolution of the use of artificial intelligence (AI) in the intellectual property field. One area that we saw potential in was AI for patent searching. To learn more, we decided to run tests that could help us understand how AI-powered search tools compare to the industry standard Boolean approach. These tests aimed to investigate the impact of different search technologies on result comprehensiveness and the time required to find relevant results.
In previous published reports, we observed that AI tools overall performed better than a Boolean-only approach. However, we also found that performance was uneven. While AI-powered tools did achieve higher overall scores when averaging across all test cases, they did not outperform Boolean on every case. In a few cases, the traditional method was significantly better. Since publishing that report we’ve heard more and more that it is becoming standard practice to start a search with an AI tool and finish with a Boolean tool. Combining approaches certainly makes sense given that we saw a wide variation in performance across different methods.
We recently tested Amplified AI and were surprised at what we found. Amplified turned out to be the most accurate method. What was particularly surprising was that Amplified scored higher than the other AI tools both when averaging across all cases and when comparing head-to-head on each of the ten individual test cases. Amplified also performed better on every case when compared to the traditional search method. This was the first time we observed consistent high scores from an AI-powered tool.
STUDY RESULTS
The average normalized score of Amplified was 0.745 across all the tests, while the other AI-powered platforms scored, on average, 0.626 – which represents an overall 19% increase in comprehensiveness.
Amplified’s highest scoring case was 0.900, a 9.5% to 19% increase over the highest score from other methods. On the other hand, Amplified’s lowest score was 0.533 which is 14% to 33% better than the lowest scores from other methods.
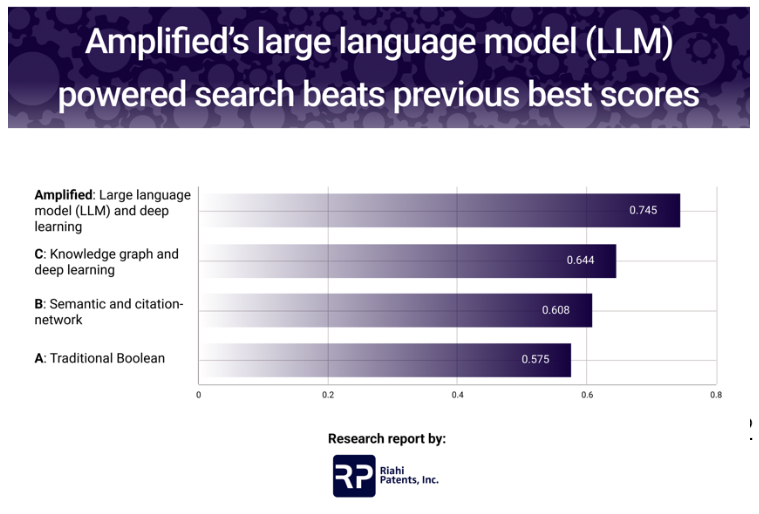
Looking at the distribution of performance across cases reveals that Amplified also delivered consistently high scores across the range of cases with particularly strong results in the top three quartiles.
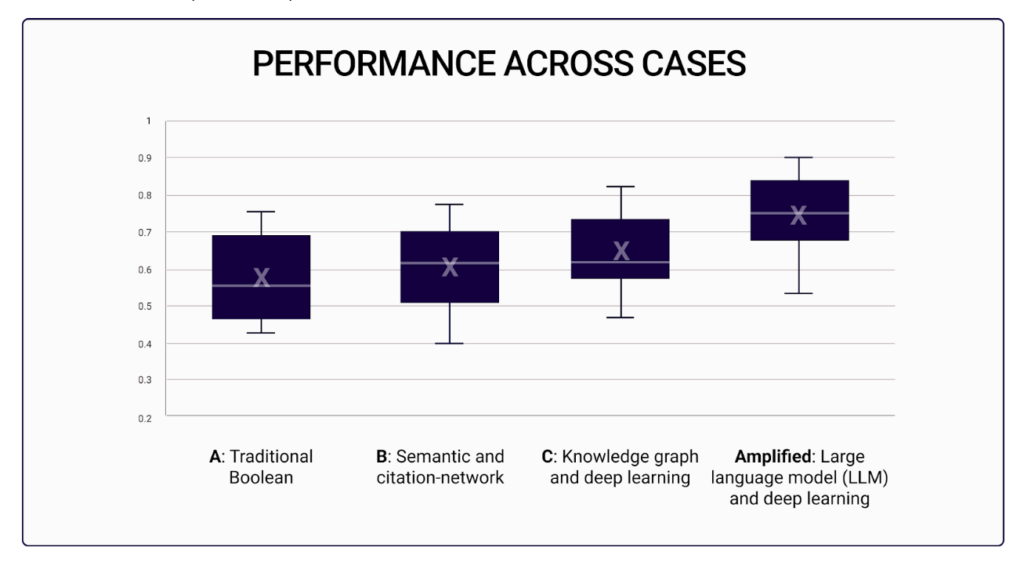
In this study, Amplified achieved higher quality than previously possible in all cases. This certainly seems like a significant advance in the field – with implications for patent search and, more broadly, patent quality. Among other conclusions drawn from the experiment, Riahi Patents can confidently report that Amplified delivers superior performance compared to traditional Boolean search and other popular AI-powered search tools in the market.
TECHNOLOGY AND PRODUCT REVIEW
Why did Amplified perform so well in this study? While we can’t answer that question definitively, we did observe that there are two things that Amplified AI appears to do differently compared to other tools.
First is the AI that they use. The tools that we’ve examined cover a range of technologies. Method A relies solely on advanced Boolean logic and complex queries. Method B takes a more traditional AI approach that relies on semantic understanding and leveraging citation networks. Method C is on the cutting-edge and uses knowledge graphs to represent patent information then refines that with deep learning. Amplified builds on the large-language model (LLM) branch of AI development.
LLMs are the same technology that the now ubiquitous ChatGPT is built on. We note that the EPO has also publicly shared that they build on LLMs in combination with a variety of other machine learning techniques. In the EPO’s case, they originally built using an LLM called BERT but have since switched to a different LLM model called RoBERTa which they say yielded a significant improvement. This shows that while LLMs in general are powerful, they are not all the same. Different choices in which language model to build on lead to very different outcomes. Amplified publicly states that they have trained their own LLM custom-built for working with long, complex, technical documents like patents.
The second thing that Amplified does differently is how they allow users to combine AI and Boolean tools in one place. As mentioned above, we’ve heard that it is quickly becoming standard practice to start a search in an AI tool and then finish with a Boolean tool. So perhaps it is not surprising that Amplified did well in our tests given that they have combined the two techniques in one tool. As users we certainly appreciated the feeling of control from having both options available.
In conclusion, we were impressed with Amplified’s AI accuracy and well-designed product. If you are using or evaluating AI tools, we recommend giving them a try. We also note that Amplified recently joined WIPO’s Inventor Assistance Program as a sponsor and are happy to see such a powerful tool used to give back to the community.
METHODOLOGY
Patent search result quality is notoriously difficult to measure due to a variety of variables like analyst experience, subjectivity, and time spent. To design our study, Riahi Patents developed ten test cases based on non-provisional patent applications and asked trained patent analysts to perform a comprehensive prior art search using a selection of AI-powered tools and traditional search methods. All the tools were used for all ten cases.
To control for experience, analysts were randomly selected from a pool of professionals with the same level of experience. Time was held as a constant so that quality could be compared on an apples-to-apples basis. Analysts were each given four hours and required to use the full time allotted to return the ten most relevant prior art results possible.
To support this methodology, Riahi Patents also used a normalized scoring system so that search result quality could be compared. Each of the ten cases had between eight and ten features that needed to be addressed – partially or totally – by the prior art found. After spending four hours on each case, the analysts returned the results to Riahi Patents, where they were then analyzed by an independent team of patent analysts to verify and ensure the quality of the tests. Based on the number of features found, each search tool received a score ranging from zero to one. For example, in a test using tool “X” where four out of eight features were found, the tool “X” would receive a score of 0.500 for that particular case.
ABOUT RIAHI PATENTS
Thank you for reading. Studies like this require a lot of time and effort to design and conduct. Nonetheless, we think they are valuable ways to give back to the IP community and help us to constantly improve and evolve. Knowing what tools are available and how to best use them is critical to ensuring that we can get the right information in a cost-effective way and support clients with the right advice for their situation. If you’d like more information on our AI studies or anything else please get in touch.
Hello There. I found your weblog the use of msn. That is a very neatly written article.
I’ll make sure to bookmark it and return to learn extra of your helpful information.
Thanks for the post. I’ll definitely return.
I appreciate you sharing this blog.Really looking forward to read more. Fantastic.
Thank you for some other wonderful article. Where else may just anybody get that kind of information in such a perfect method of writing? I’ve a presentation next week, and I am on the look for such info.